The RNA structure presents unique challenges for computational models.Credit: Getty
At a virtual conference in November 2020, the winner of a biennial protein-structure-proclamation challenge was announced: Alphafold. Manufactured by Google Deepmind, this computational tool had solved its rivals by solving dozens of protein structures with nuclear level accuracy, completing an achievement by the researchers who had tried for decades.
The challenge known as the Critical Assessment of the Protein Structure prediction (CASP) was launched in 1994 from their amino-acid sequences to pursue a computational tool for 3D protein configuration modeling. Teams of scientists put their computational models against each other, which was already trying to generate the most accurate predictions for unknown protein structures, which is experimentally resolved using methods such as X-ray crystallography and cryo-electron microscopy before the incident.
Alphafold’s 2020 predictions rivals those solved with these tried and tested techniques, and have become a favorite of the structural-biology community ever since. Its repository – alphabet protein structure database – includes some 200 million structures, and, in 2024, Alphafold’s developers shared half of the Nobel Prize in Chemistry for their work.
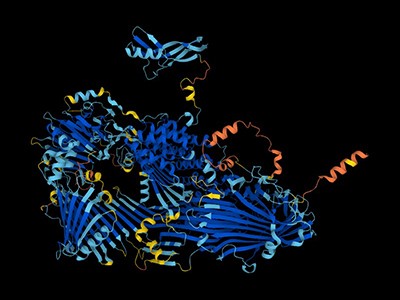
‘The Sampoorna Protein Universe’: AI predicts almost every known protein size
But it is protein. In 2022, the CASP organizers paid their attention to the square of a separate, yet challenging, biomolecule: RNA.
With protein, to determine the RNA structure, usually expensive and time -consuming experimental methods are required. Computer tools can help, but RNA is a hard walnut to crack. A simple reason, according to U Li, is a computer scientist, historical at the Chinese University of Hong Kong. For a long time, most scientists did not think that RNA was quite interesting to study biology. But RNA also produces unique molecular challenges, and relatively low data is available to train computational models that perform so well with proteins.
However, researchers are becoming creative, and there is an increasing toolkit of computational devices emerging to help predict the RNA structure. Many of these Artificial Intelligence (AI) include the latest developments, including large language models (LLMs), which outlines popular chatbots such as chatgips.
“RNA folding is a very difficult problem,” accepts a computational biophysicist X-J chain at Missouri University in Colombia. But AI, he says, getting “better and better”.
Elusive target
For a long time, RNA was seen as a mediator only between two and interesting classes of the molecule: DNA, ‘Jeevan’s blueprint’, and protein, ‘building blocks’ of the cell. Only a small fraction of human genome encounters the protein, yet most part of the non-coding genome is transferred to RNA. In the last few decades, scientists have discovered that these non-coding RNAs mediate essential functions in healthy cells-and contribute to many diseases.
How these RNAs work, in many cases, a mystery. Researchers hope that, by determining their size, they will be able to understand the better role that these molecules have a question of our cells – a question of form dictating function. “In biology, we believe that the sequence structure is very likely to determine, and that the structure is very likely to determine the task,” Lee says.
But computational equipment is behind their protein counterparts to predict the RNA structure. Even Alphafold3 decreases when the latest version of the structure-future device of the lamp-rhetoric-RNA.
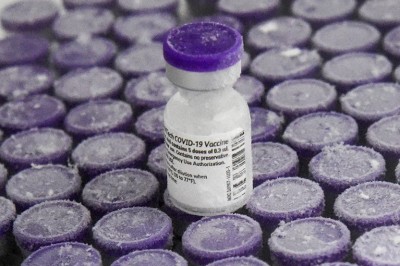
‘Remarkable’ AI Tool Design MRNA vaccines which are more powerful and stable
“If you recently look at CASP competitions, we are at the point where, on the protein structure side, completely automatic team is as good as human teams,” called a system biologist at the University of Lidia Freddolino, Michigan, and a scientific advisory board member for Circinova, a company, a company that uses a company that uses deeper. “For RNA, we have nowhere – all top groups use human intervention heavy.”
RAN-structure predicted in CASP competitions in 2022 and 2024, and Freddolino participated in both. In the latest phenomenon, the first place team, CASP16 used a hybrid approach to predict RNA structures: AI a defined, combined with physics-based algorithm. According to Chen, who led the winning group, used the Alphafold3 for the first time to generate a potential RNA structures, and then applied a physics-based model that examines the ‘energy landscape’ of potential structures that are most likely to indicate confirmation. (Chen’s team has licensed their software to many biotechnology firms.)
Researchers developing AI-cavalry equipment to predict RNA structure faced many obstacles. One is that RNA molecules have characteristics that make their structures difficult to predict naturally. RNA molecules have more flexible backbones than proteins, and their structures are more dynamic, which means they can undergo adequate changes when performing their biological functions.
At the top of it, RNA molecules lack various chemistry that can be found in proteins, such as acidic and basic relics, which allow to create a stable connection. Instead, the sections of RNA interact in all types of “strange and amazing ways”, Freddolino says, such as different -base pairing and metal ions through participation. As a result, microscopic variations between the best and worst models are difficult to spot the protein.
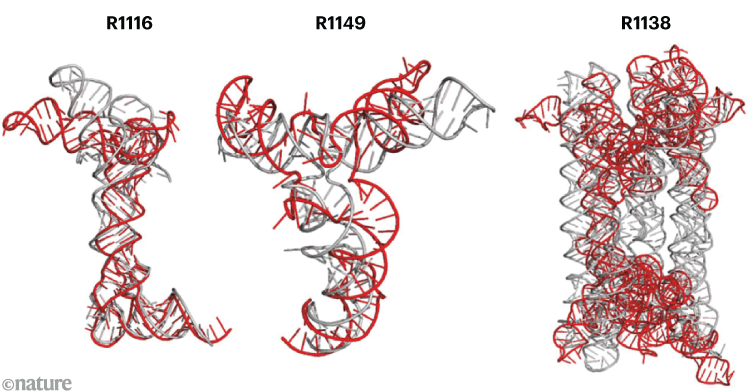
Natural (R1116 and R1149) and synthetic (R1138) are used in structures, composition-perdextion tasks CASP15, experimentally measured (gray) and predicted using AI tools (red).Credit: W Wang Et al,temperament,
The chemical alphabet of RNA is also difficult to interpret: the four chemical base making RNAs are less different than the 20 amino acids found in proteins. This means that each RNA base has less information than amino acids. One of the reasons such as the alphafold has been very successful, freddolino notes, the ability to use large sequence databases to indicate the pattern of interaction between various amino acids – and it is much more difficult to do with RNA.
And then there is a lack of known RNA structures. Protein data banks, a reserves of 3D macromolecular structures have approximately 200,000 protein structures and less than 2,000 RNA. This deficiency of data means that the AI-based structure has less information to feed the algorithm that reduces prediction.
“We can do what we can do with limited data,” says Jim Collins, a biomedical engineer at the Massachusetts Institute of Technology at Cambridge. “The region will move ahead with collection and cursoring of many more structures.”
Bring in
Researchers are working to solve these challenges, and in recent years, many AI-based RNA-structure-future equipment have emerged. Prior to 2020, most ways to predict the RNA structure were based on algorithms defined by specific physical or mathematical models, according to Jiyani Yang, a computational biologist at Shedong University in Kingdao, China. But the success of Alfafold has inspired people in the RNA sector to implement AI for this problem, they also say.
Yang and his colleagues designed the AI tool, Trroseturna, completely automated (and independently available), which combines deep learning with elements of Rosetta, a computational tool that is used to determine molecular structures, designed by David Baker at Washington University in Seattle, who was composed of alphabets of alfold Had shared together.
For example, for protein, RNA structure is at several levels: nucleotide sequence (primary); The intermediary structures that find the base pairs find their complement (secondary); And final, 3D structure (tertiary). RNAs can also make premises with each other and other molecules (Chaturdhatuk). First, Trosettarna produces predictions of primary and secondary structures, then, with the help of a classical physics-based model, it rebuilt the tertiary structures. Secondary structures-as ‘hairpins’ which are formed with each other as the small segments of the sequence pair-are more important for RRNA, as they are for protein, Yang says, and the use of in-in-in structures is one of the key to the success of this model.
Yang’s team raised and found trochetarna against other automated equipment and found, based on an assessment with two independent data sets of dozens of RNA, that it crossed those devices in accuracy.1In 2024, the software finished fourth in the CASP16.
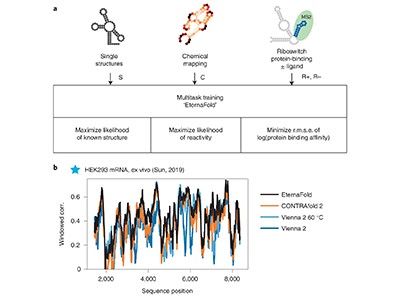
RNA learning from crowded RNA learning the prophecy of RNA structure