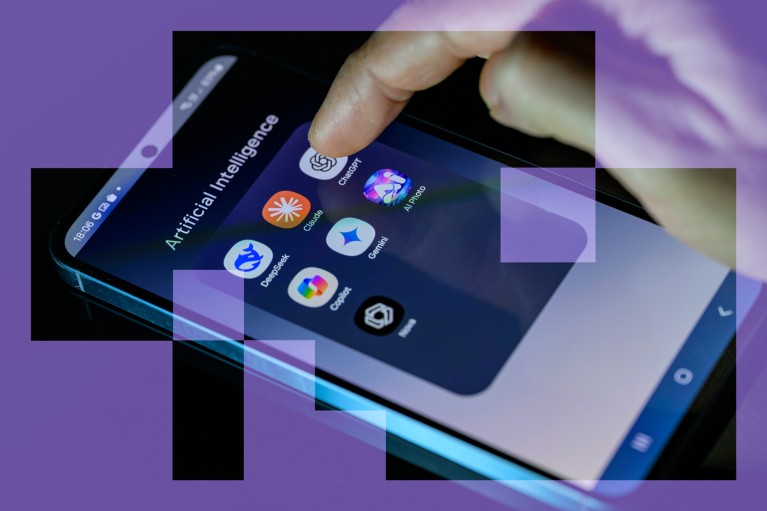
A new metric assesses the progress of the AI model.Credit: Jonathan RAA/Nurphoto/Getty optimized
Today’s Artificial Intelligence (AI) systems cannot beat humans on long tasks, but they are improving rapidly and according to the analysis of leading models, can soon shut down the difference compared to many anticipated.1,
Berkeley, a non-profit organization METR in California, created about 170 real-world functions in coding, cyber security, general logic and machine learning, then established a ‘human baseline’, measuring how much time it took to the expert programmer to complete them.
The team then developed a metric to assess the progress of the AI model, which it calls ‘task-completion time hoarisan’. This is the time when programmers usually take to complete the tasks that the AI models can complete at a certain success rate.
In a preprint posted on ARXIV this week, METR reports that an early major language model (LLM) GPT-2 published by Openai in 2019 failed on all the tasks that took human experts for more than a minute. Cloud 3.7 sonnet released by the US-based start-up anthropic in February completed 50% of the works, which will take people 59 minutes.
Overall, the time of 13 major AI models has almost doubled every seven months since horizon 2019, the paper is detected. In 2024, the exponential growth of the AI time horizon accelerated, the latest model almost doubled its horizon to almost every three months. The work has not been formally reviewed by the colleague.
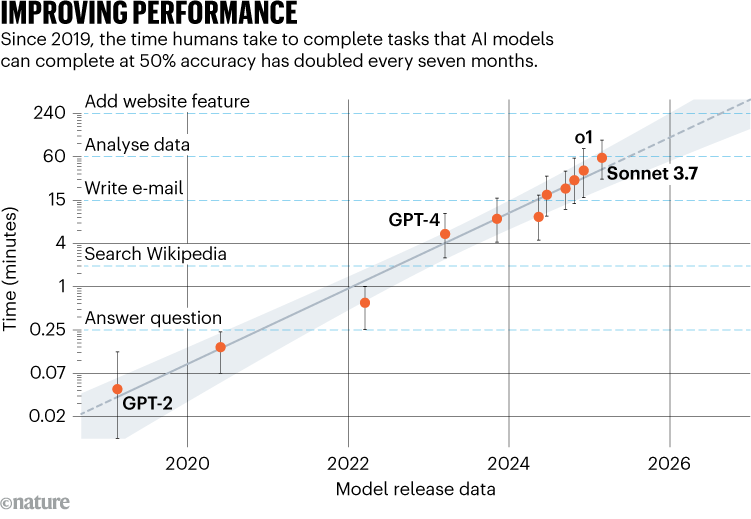
At the rate of progression of 2019-2024, METR suggests that the AI models will be able to handle the tasks that humans take 50% reliability by 2029 in a month, possibly soon.
A month of dedicated human expertise, paper notes, can be enough to start a new company or scientific discovery, for example.
But Professor Joshua Guns, a management professor at the University of Toronto in Canada, who wrote on AI’s economics says that such predictions are not useful. He says, “Extraplation is attractive, but still much that we do not know about how AI will actually be used to be meaningful,” they say.
Human vs. ai assessment
The team chose 50% success rate as it was the strongest for small changes in data distribution. Co-writer Lawrence Chan says, “If you choose too little or too much threshold, removing or adding a successful or single unsuccessful task respectively, changes your estimates a lot,” co-writer Lawrence Chan is called Lawrence Chan.
The average time horizon was reduced by a factor of five by increasing the reliability range from 50% to 80% – although the overall double time and trendline were similar.
In the last five years, improvement in general capabilities of LLM has been operated by large scale increase – the amount of training data, training time and number of model parameters. AI’s logical arguments, equipment use, error improvement, and improving self-awareness in functioning mainly makes paper progress on time horizon metrics.
Metr’s time-Horizon approach addresses some limitations in the current AI benchmark, which map to the real world, only relaxed and quickly ‘saturated’ because the model improves. It provides a constant, spontaneous remedy that better capture better long-term progresses, called co-writer Ben West.
In the West, it is said that the AI models receive supernatural performances on many benchmarks, but have had a relatively low economic impact. West says that the latest research of METR provides a partial answer to this puzzle: the best models sit on the horizon of about 40 minutes, and at that time a person does not have a much more economically valuable work that can do at that time.
But San Francisco, an AI researcher and entrepreneur in California, Anton Trionikov says AI would have a greater economic impact if the organizations effectively were more inclined to take advantage of the model and invest.